In our increasingly digital world, technology bridges gaps and enhances accessibility. Text-to-speech (TTS) systems stand as a testament to this evolution, transforming written words into spoken language. This article aims to unravel the intricate mechanics behind text to voice technology, exploring its evolution, key components, and the impact it has on various aspects of our lives.
Evolution of Text-to-Speech Technology:
Text-to-speech technology has come a long way since its inception. Early attempts were rudimentary, producing robotic and unnatural voices. However, with advances in artificial intelligence (AI) and natural realistic ai Voices language processing (NLP), modern TTS systems have achieved remarkable improvements in both quality and naturalness.
Speech Synthesis Methods:
Concatenative Synthesis: In the early stages, concatenative synthesis involved stitching together pre-recorded segments of human speech. While it provided better naturalness, it had limitations in terms of flexibility and adaptability.
Formant Synthesis: This method used mathematical models to simulate the human vocal tract. Though computationally intensive, it allowed for more control over the generated speech.
Statistical Parametric Synthesis:
Hidden Markov Models (HMMs): HMMs were employed to model speech patterns, but they faced challenges in capturing the richness and diversity of natural speech.
Deep Learning Approaches: The advent of deep learning, especially recurrent neural networks (RNNs) and long short-term memory networks (LSTMs), revolutionized text to audio. WaveNet, developed by DeepMind, and Tacotron, a sequence-to-sequence model, exemplifies the power of deep learning in generating natural-sounding speech.
Key Components of Text-to-Speech Systems:
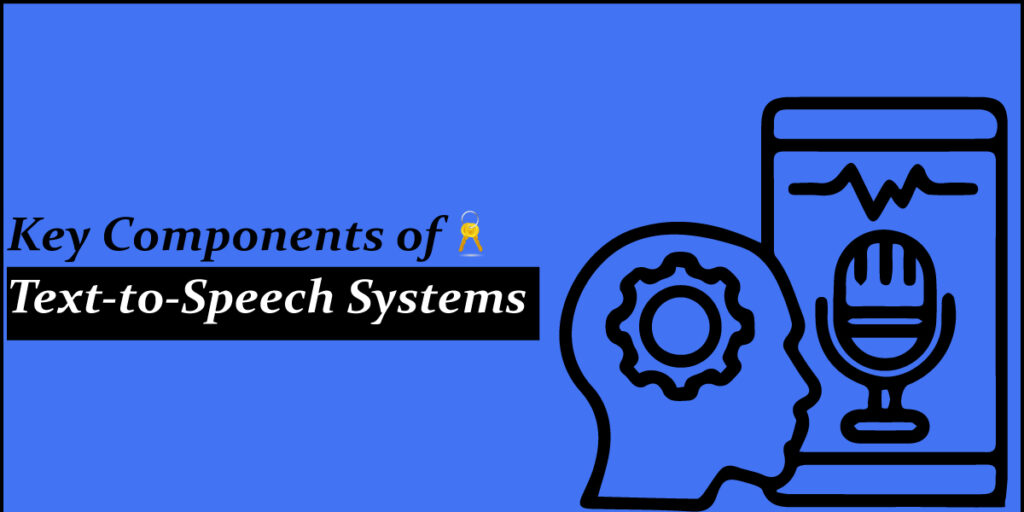
Text Analysis:
Text Preprocessing: The input text into text to voice converter undergoes preprocessing to handle punctuation, special characters, and formatting. Tokenization breaks down the text into smaller units, such as words or phonemes.
Prosody Modeling: Prosody, encompassing pitch, rhythm, and intonation, is crucial for natural-sounding speech. Read text aloud systems and analyze the text to determine appropriate prosodic features.
Phoneme Mapping:
Grapheme-to-Phoneme Conversion: Converting written text into phonetic representations is essential for pronunciation. Grapheme-to-phoneme models map written symbols to corresponding speech sounds.
Acoustic Modeling:
Deep Neural Networks (DNNs): Acoustic models, typically implemented using DNNs, learn the relationship between linguistic features and corresponding audio representations. This enables the system to generate realistic speech waveforms.
Waveform Synthesis:
Concatenative Synthesis: In modern systems, concatenative synthesis has evolved, with units being selected dynamically based on context. This enhances the overall naturalness of the synthesized speech.
Generative Models: Recent advancements in generative models, such as GPT (Generative Pre-trained Transformer), have been applied to read text aloud. These ai voice generator models can generate high-quality speech waveforms directly from text, eliminating the need for separate acoustic models.
Impact on Accessibility and Human-Computer Interaction:
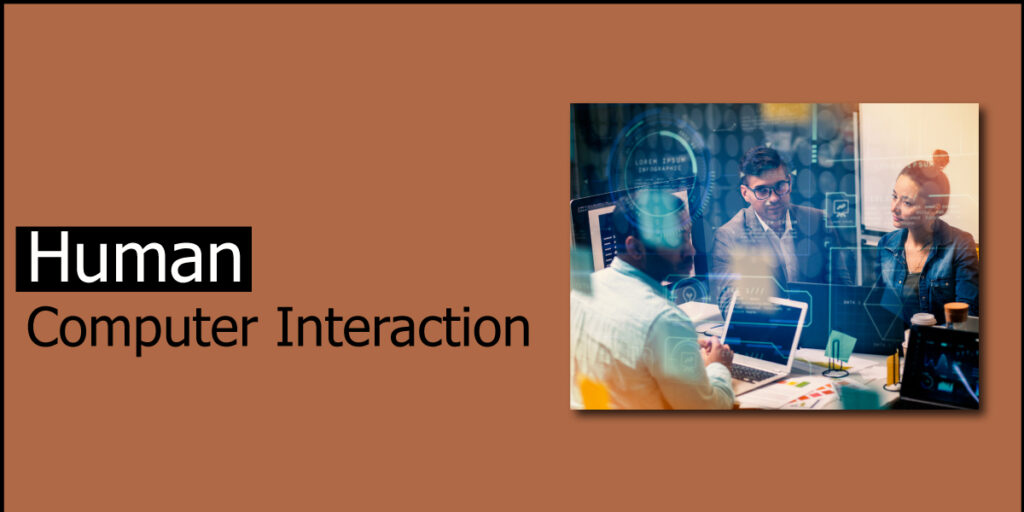
Accessibility for Diverse Audiences:
TTS text to audio converter systems play a crucial role in making digital content accessible to individuals with visual impairments. Screen readers leverage Text to speech to convert written text into spoken words, enabling visually impaired users to interact with digital information.
Multilingual Support:
AI voice generator technology has facilitated the development of multilingual systems, breaking down language barriers by providing a voice to written content in various languages. This is particularly valuable in global communication and language learning applications.
Humanizing Virtual Assistants:
Virtual assistants, powered by Text to speech systems, have become integral parts of our daily lives. The ability to produce natural-sounding speech enhances user experience, making interactions with devices like smart speakers and chatbots more engaging and human-like.
Challenges and Future Directions:
Emotional Expression:
While Text to speech systems have made significant strides in naturalness, incorporating emotional nuances in speech remains a challenge. Future developments may focus on infusing synthesized speech with appropriate emotional cues for a more immersive experience.
Real-Time Adaptation:
Achieving real-time adaptation to context and user feedback is an ongoing challenge. Systems that can dynamically adjust prosody, pace, and style based on context and user preferences are areas of active research.
Conclusion:
Text-to-speech systems have evolved from basic concatenative synthesis to sophisticated deep-learning models, revolutionizing the way we interact with digital content. The fusion of AI, NLP, and advanced neural network architectures has propelled text to sound technology to new heights, enhancing accessibility, enabling multilingual communication, and humanizing virtual interactions.